Racial Bias, Data Science, and the Justice System
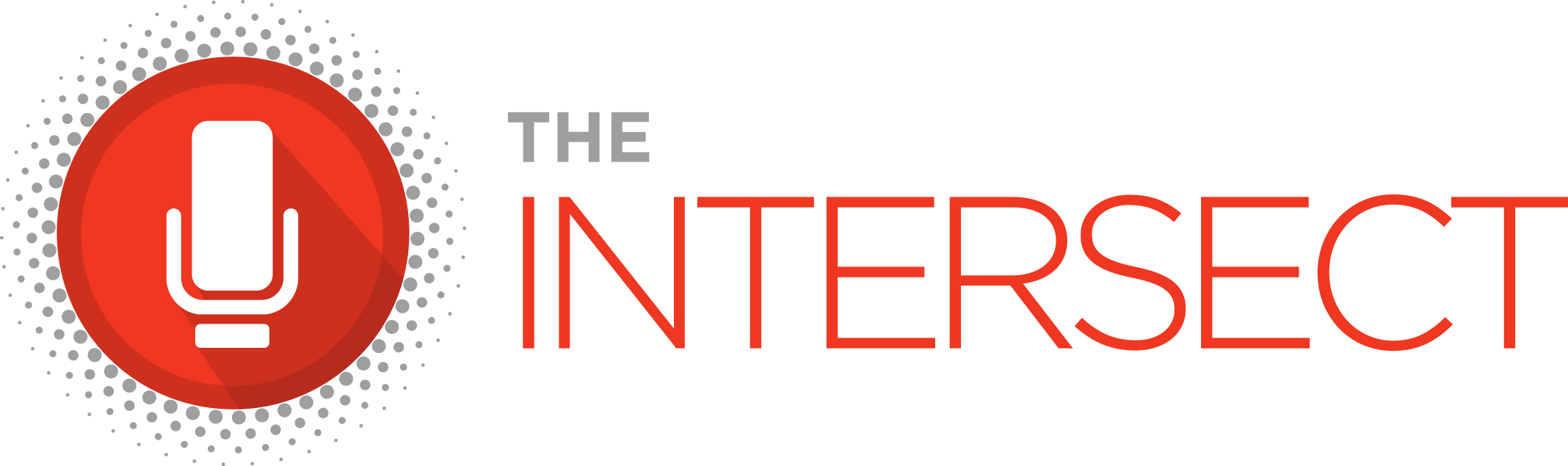
Challenges in areas ranging from education to the environment, gender to governance, health to housing don’t exist in a vacuum. Each month, Abt experts from two disciplines explore ideas for tackling these challenges in our monthly podcast, The Intersect. Sign up for monthly e-mail notifications here. Catch up with previous episodes here.
From pretrial detention to sentencing to community supervision, data can inform and guide how our justice system works but, for better and for worse, that data doesn’t exist in a vacuum. Holly Swan and Sharmini Radakrishnan discuss the approaches we can take—and the expertise we’ll need—to eliminate racial bias in the justice system.
Read the Transcript
Eric Tischler: Hi, and welcome to The Intersect. I'm Eric Tischler. Abt Global tackles complex challenges around the world, ranging from improving health and education, to assessing the impact of environmental changes. For any given problem, we bring multiple perspectives to the table. We thought it would be enlightening and maybe even fun to pair up colleagues from different disciplines so they can share their ideas, and perhaps spark new thinking about how we solve these challenges.
Today's a little unusual. I'm alone in Abt Studio One with engineer John and our colleagues, Holly Swan and Sharmini Radakrishnan, are calling in from our offices in Cambridge, Massachusetts. Holly is a sociologist and implementation scientist with expertise in criminal justice and behavioral health. She's directed several mixed-methods evaluations of practices and initiatives within the justice system. Sharmini is an economist specializing in quantitative research methods for health and criminal justice. She's analyzed and validated risk assessment tools used by federal and state criminal justice agencies. Thank you both for joining me.
Holly Swan: Thank you for having us.
Sharmini Radakrishnan: Thank you.
Eric: So, Holly, I came to you with an idea for this podcast, because I'd read about the challenges of applying AI and machine learning to the justice system sentencing procedures, but you said there are potential applications throughout the justice system. Can you tell us what we're trying to solve for, with these technologies?
Holly: Sure. Thanks, Eric. Yeah, I think, the idea of artificial intelligence and machine learning as applied to criminal justice is relatively new. Although, the problems that it's trying to solve are pretty old. So thinking about the justice system as a continuum, there's many points along the way, from pretrial all the way through re-entry after somebody serves time, onto community supervision even, where decisions need to be made. And at each of those decision points, there is a possibility that bias gets introduced into those decisions based on a variety of factors.
So, I think the use of standardized tools and assessments is something that is currently occurring in the criminal justice system. And the idea of using technology to help make these decisions more objective and try to reduce the bias that goes into the decision making, is what the appeal is around using data science. I think there are some risks to it as well that we'll probably get into today, but that's the overall thinking around this idea.
Eric: Got it. And so, Sharmini, do you want to talk a little bit about what technologies and approaches we might want to take to address both these concerns and the benefits of these technologies?
Sharmini: Sure. I'll start off talking about what a risk assessment is a little bit more, and then we can talk about some of the challenges of using them. So a risk assessment is a tool that uses an algorithm to predict an individual risk of reoffending. And that risk prediction is used at different points in the criminal justice system to inform—it could be the sentence that they receive or the supervision conditions that someone receives when they're coming out of prison.
So, risk assessments are a tool that use an algorithm to predict an individual's risk of reoffending. And that risk prediction that's generated by the assessment is used at various points in the criminal justice system to either inform the sentence that someone receives or the supervision conditions that someone might get when they're released from prison. And it's typically not the only thing that's influencing the sentence or the supervision conditions, but it's a factor. Increasingly, I think, in most agencies, some type of assessment is being used.
And the types of factors that get included in these models are things like criminal history, education, employment, substance use, antisocial behavior, and pro-criminal attitudes. So the way these tools are developed, is that the developer starts with some sample of individuals that ideally represents the target population that you're trying to assess.
Eric: Sorry, by target population, what do we mean in this context? How are we defining that?
Sharmini: So for example, if it's people who are being released from prison and going out into the community, then they would start with a sample of individuals like this.
Eric: Great.
Sharmini: And then they essentially apply a predictive model to these individuals. Where the predictors are the factors that I just mentioned, criminal history and various other things. So the stronger the predictor is, the greater the weight that it gets assigned in this algorithm. And so that's how these tools are developed.
A challenge with these assessments of course is that they're developed using data, and the data on criminal history reflects not just the individual's behaviors and choices, but also decisions made by police officers, prosecutors, and judges. And so to the extent that there is some bias in those decisions, the data will reflect that bias. And of course the algorithm then replicates that bias, because the algorithm is only as good as the data underlying it.
So that's, I'd say, the main challenge with risk assessments that I think has been highlighted a lot recently in the media, that's seems to be the key issue.
Eric: We're trying to combat biases, like the idea that the technology and the data, rather, really can help us look past biases, but the struggle is to remove the biases from the data in the first place, from that sample.
Sharmini: Exactly. I mean, that's exactly right. I think just to put things in context and, really, Holly already mentioned this. Before risk assessments were used, the agencies were relying on almost purely subjective judgments from law enforcement officers and judges. And so the intention of risk assessment is really to introduce a standardized approach to reduce bias, or ideally remove bias. But as you've said, it's the data itself does reflect that bias.
Eric: We're trying to correct for bias, but what is the effect and impact of bias? Why do we need to correct for it? I guess let's answer that question, and then talk about what are we doing to address these challenges.
Holly: Industry officials are trying to figure out where to devote their resources, and trying to figure out how best to both administer justice, but also prevent future recidivism, criminal activity, and keep the community safe. And then, in addition to that, we have this new problem of mass incarceration, and we're trying to reduce the number of people in prison who maybe don't need to be. And so one of the ideas is that by eliminating bias, we can administer justice more fairly, but then also reduce the prison population by keeping people in the community who maybe don't need to be incarcerated.
I think also the idea of risk assessment in particular, so there's a framework in criminal justice, the risk, needs and responsivity framework, or RNR for short, which basically says you should focus your resources on people at the highest risk for failing on supervision or for committing new crimes. And of course, there's bias that goes into that as well. We have assumptions about who might be more likely to recidivate or to commit criminal crimes. And by reducing those biases, and instead of formulating it again on something more objective, criminal history or other criminogenic thinking type of things that are evidence-based, then those decisions are more appropriate for making decisions to focus resources on those people.
Eric: Right. Okay, great. And so what are we doing to help get to that point, to help sanitize that data or level those perspectives?
Sharmini: So, one thing that Abt does is we do independent validations of risk assessments. I think that is one of the most important things that has to be done with effective risk assessments, it's what a validation study is, is that it assesses the accuracy of the instrument by comparing the predicted risks to the actual outcomes that we would observe in the individuals who are given the assessment. And I think independent validation studies that are done by a third party that is not affiliated with the developer of the instrument, and not affiliated with the agency that is administering the instrument, is really important. And Abt has done those studies, both for federal agencies and for state agencies. And with these assessments, you look at the accuracy of a tool and you can look at subgroups as well, to the extent that you have a large enough sample, to see how these error rates look like for the overall population and also for the different subgroups that you're interested in.
I'd say that's, I think, the biggest thing, but also, we've seen in our work where we do some qualitative research as well, the implementation of these tools is really important, and having the staff get the right training to be able to use the tools correctly, is also really important. I think training and retraining, regular retraining, is really important to make sure they're actually using the tool as intended.
Holly: Yeah. And I'll just reiterate that a little bit. I think, so in addition to validating tools that agencies are developing for their specific agencies or populations, there are existing actuarial risk assessments out there that have been demonstrated to have some validity already. And so that next step of getting those implemented—and getting them used appropriately—is really important. Rather than reinventing the wheel with new tools, maybe we could figure out, okay, does the application of this tool in this context work?
Eric: So, that's what I was going to ask, what are the next steps? We know what the process is, or what we need to do to make these tools more effective. What do we do about getting them implemented so we can have that more balanced approach to the justice system?
Sharmini: I think some of the things we've mentioned are really ongoing things that need to happen regularly. But I think aside from that, I would say, getting stakeholder input at the time that any tool is implemented, is really important. Because sometimes these tools are developed by a company that is in a different location and the sample they've used to build a tool is a very different sample from the target population that a criminal justice agency is using in another part of the country. They may be just aspects of the tool that don't always translate. And so their officers, or whoever is administering, may just not be using it correctly for that reason. Sometimes the wording of the questions are they may not really be understanding it, and then recording the answers accurately. And so, getting that input ... Again, this all goes back to implementation, how important implementation is. It's really important in that regular retraining, I think.
Holly: And I'll add, in addition to training on using the tool, I think training in capturing quality data and reliably captured data that's consistently collected in order to be used appropriately. And I think a challenge there that agencies face, and another area that I think machine learning and AI can help with, is a lot of agencies collect data through case notes. And so it's just written texts and not discrete fields, that maybe it can be input into a statistical program, for example, to make predictions. And so something that machine learning and different technologies can use to take that, all that body of narrative texts and turn it into some sort of discreet categories, would be a way to move the field forward, I think.
Eric: Very cool. And that's stuff that Abt does, isn't it?
Holly: It sure is. We have a great team that works on that.
Eric: That seems like a great place to end. Thank you both for joining me.