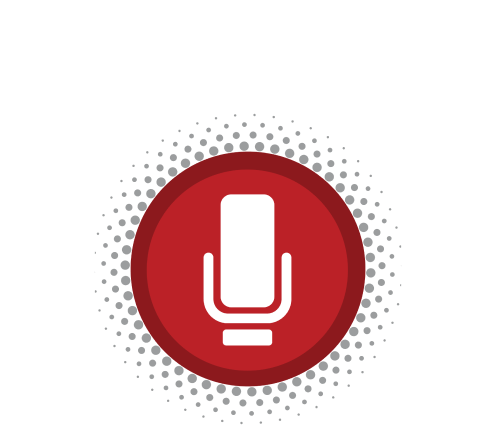
Defeating TB: Private Sector to Predictive Analytics
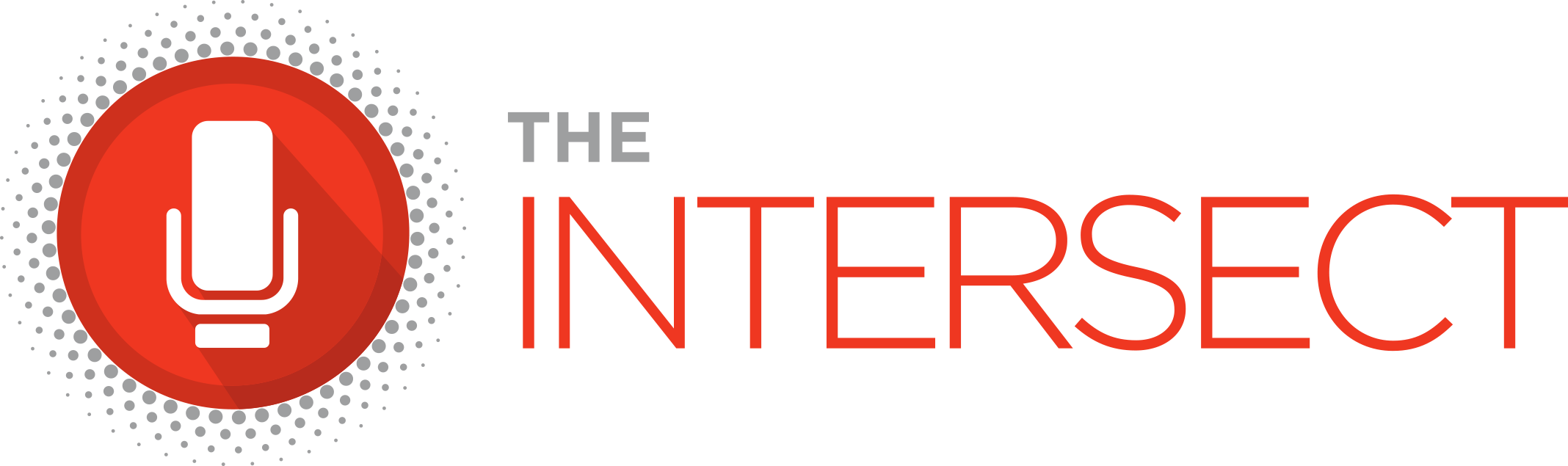
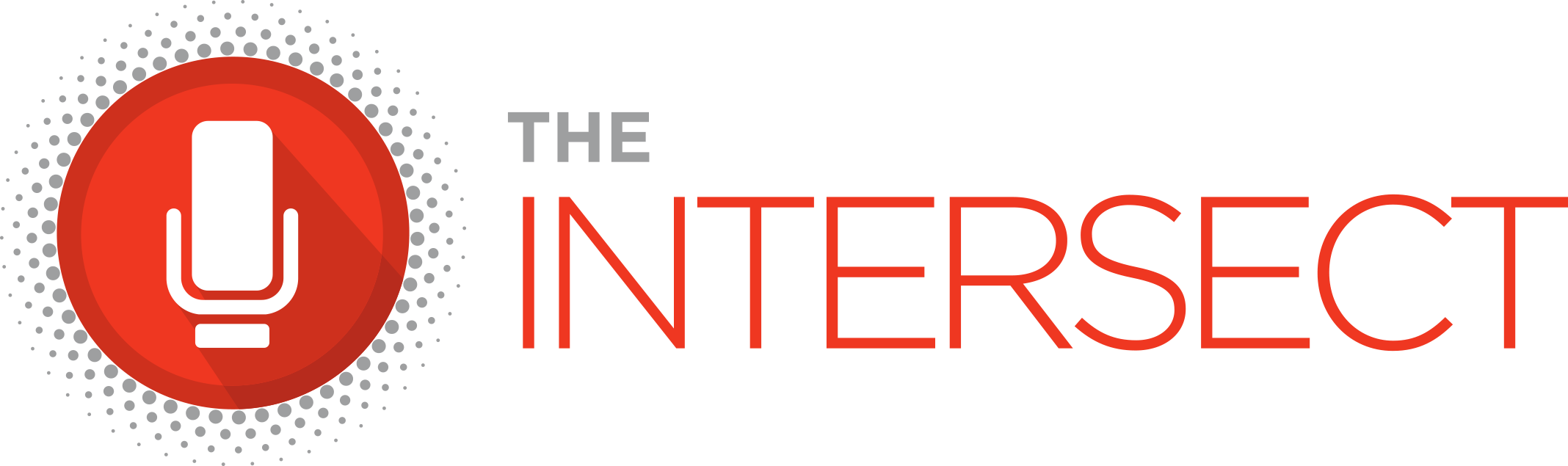
Challenges in areas ranging from education to the environment, gender to governance, health to housing don’t exist in a vacuum. Each month, Abt experts from two disciplines explore ideas for tackling these challenges in our monthly podcast, The Intersect. Sign up for monthly e-mail notifications here. Catch up with previous episodes here.
How do we defeat tuberculosis? With sustainable solutions. With support from the private sector. With data.
Abt’s Elaine Baruwa (SHOPS Plus’s Nigeria tuberculosis project manager) and Jason Brinkley (leader of our digital solutions research, design and analytics group) break it down in the latest episode of The Intersect podcast. From data visualizations to predictive modelling, they discuss tools and strategies to increase—and improve—private sector involvement and to get ahead of drug-resistant TB. Listen:
Read the Transcript:
Eric Tischler: Hi and welcome to the Intersect. Today I'm joined by Elaine Baruwa and Jason Brinkley. Elaine has more than 15 years of experience conducting program evaluations, economic evaluations and working on pricing and costing policies and tools for financing health services and health systems in Nigeria, Haiti, Ethiopia, China, Bangladesh and other low income countries. And she's been managing USAID's SHOP Plus Nigeria Tuberculosis Program.
Jason is a biostatistician data scientist and health researcher. He leads Abt’s research design and analytics group, which focuses on sampling, survey weighting and statistical modeling. He has expertise in a wide range of analytic methods and he specializes in machine learning, customized data visualizations, maps and dashboarding. Thank you both for joining me.
Elaine Baruwa: Happy to be here.
Jason Brinkley: Glad to be here.
Eric: Elaine, why does tuberculosis continue to be an issue in developing countries?
Elaine: Where you have economic growth, you have growth of urban cities. And the poor people that come to those cities or arrive in those cities tend to live in slums. Slums are the perfect place to have tuberculosis. You live in close contact with other people and it's an infectious disease and you probably don't have great nutrition, etc., or even access to good quality healthcare. So it's a great place, a growing city, to have tuberculosis. So the countries where we work on tuberculosis [through] USAID's SHOPS Plus Program—India and Nigeria—have large, urban, densely populated cities. In Nigeria in particular, part of the reason we have a tuberculosis burden of disease that's so high is because the system isn't strong enough to identify TB cases. So what we have is proximately 400,000 people getting TB each year. Only a hundred thousand or so of those get identified and placed on treatment.
So the other people don't get treatment and remain infectious to other people. The reason people don't get identified is because the providers themselves are not great at screening for TB. So we have what we call a low index of suspicion. People don't think it's TB so they don't test for TB.
The second reason is that a lot of the TB program where support has been given before has been focused on the public sector, not the private sector, but in a country like Nigeria, 70 percent of all health expenditures occur in the private sector. That's where people go first. So if those providers have a low index of suspicion for TB, it means people go in, get antibiotics for coughs repeatedly but don't get tuberculosis testing and don't get treated for tuberculosis.
Eric: So it sounds like there's a data gap.
Elaine: You could say there's a data gap. There's a knowledge gap for sure. And then if the private sector is treating TB, it's not getting reported to the national TB program. So we don't know where there are hotspots of people who have TB, which means the national program can't locate them, and if we can't locate them, we can't do contact tracing, which is when we go to the household and see if other people in the household also have tuberculosis. And then we can test them and get them on treatment. So this absence of the private sector participating in the national TB program is a problem. Even after you've trained people to be able to screen for TB and treat TB, we have the issue of paper. There are so many paper registers collecting this information that it's almost impossible for the national TB program to get the information from these registers into their system so that they can then use that data to actually follow up with TB presumptive cases, TB positive cases, and do the contact tracing with their families.
Eric: Jason, when you hear this, what do you think we can be doing to help connect the dots?
Jason: There's a lot. The way I kind of see things is that there's disparities in the data, right? That there is social economic disparities, there's regional disparities, there could be cultural disparities in different areas. There's rural urban divides, things like that. All of those things are things that we can sort of measure and plot out with data potentially, right?
But there's more to it than that. I think oftentimes people think, well we just need to have data, but sometimes you might actually need specific data to tackle one challenge, another specific data to tackle the next challenge and another specific data to maybe tackle sort of an assessment challenge.
Eric: Elaine's nodding in agreement over here. What are you thinking?
Elaine: I was just thinking that Jason talked about different types of data and that's so true. So, on a typical USAID program, we're all familiar with the monitoring and evaluation data. Our MNE data, is what we collect routinely and report to our client to show that they're getting value for money with regards to whatever funding they're giving us and we are creating impact. But I ... We found on the TB program in Nigeria, that the data that you collect quarterly and you report on quarterly, it tells you what the program is doing at a very high level. But for us, we were setting the program up from scratch. These were private providers that had not previously been treating TB and had not been connected to the public sector before. That was the first thing. Secondly, we were starting out with about 1,400 private providers. So if you can picture 1,400 private providers, each of them having about six different registers, a register for screening TB, a register for diagnosing TB, a register for taking a sputum sample, it's a lot of registers, a lot of paper. And they're frankly disinclined to deal with all of that paper.
So, if we wanted them to be reporting to us, we needed to make it much, much easier. In addition to which for our own programming purposes, if we actually wanted to collect that data and use it to improve the program, we needed to be able to have that data in real time. We couldn't wait for people to be collecting data quarterly. That's 12 weeks where you can't make a change to your program, because you don't have the data. It isn't clean. So very early on in the program we decided, okay, our network officers that go out are going to have to collect this data in real time. We started off with sheets of paper and they were reporting the data and using WhatsApp. Now, we're at the stage where we actually have an app. So we're developing an app so that network officers can input information directly into the app.
We are also developing an app so that providers themselves can input information directly into the app. So that means that instead of filling out a patient's information in four different registers, they put it in the application once it's an Android app and that's it. They just have to update it. As soon as they put it in we have access to that information. So if, for example, a sputum sample doesn't make it to a lab or a result doesn't make it back to the provider, we know immediately and we can intersect. We couldn't possibly do that with paper. It had to be digital, otherwise we couldn't address those issues. This is really important to be able to turn these things around very quickly so that people can know they do or they don't have TB and they can be put on treatment quickly.
Eric: Right, right. So I've got a question for both of you. What's the next step? Elaine, I’ll ask you to go first and then Jason, maybe you can respond to ... Based on what Elaine says or maybe you have something additive.
Elaine: Well for all programs that ... USAID programs, for example, the most important thing I think is making an impact, but then secondly they have to be sustainable. So USAID has this policy of journey to self-reliance and basically it means that at the end of a program ... Actually it means that at the beginning of a program, you need to be thinking about who's going to take it over at the end of the program. And in this case it would be the government. So it's great that we've been able to use technology, but we have to be thinking about who's going to manage it when we leave.
Eric: Got you. So Jason, to you. Listening to all of this, what do you think the next step should be? What new frontiers can we be looking at?
Jason: There's a lot here. It's very much a whack-a-mole sort of thing, right? Here's a problem. It's a hotspot. It popped up, we found out about it kind of late, we're deploying a bunch of resources to sort of hammer that down or we're getting a bunch of things in place to kind of deal with that sort of threat that's already there and it's in front of us and we figured out how to measure, quantify and deal with it and assess the impact of what it is that we're doing. I think what most people are trying to look towards is getting to a place where you don't have to have that conversation of how did we not see this coming?
And I think that that is the big mandate of public health in general is to try to get to the prevention side of things. And I think there is where the promise of predictive modeling comes in. This is where I think the future is in terms of data and analytics, is that we start using all of these tools that we have available and opportunities within AI, machine learning, predictive modeling, geospatial analysis for better targeting, appending with satellite and other imagery to use other sources of information. Climate models so that we can talk about what's going on in terms of weather patterns and is that going to impact the cloistering in some of these regions?
And can we get to a place to where we're not sort of only responding to things at ... When they sort of become a full on public health crisis. How do we get to that place to where we're confident in our predictions that if we don't do something right this second or even three months ahead or six months ahead or 12 months ahead, that this is an emerging problem?
Eric: Elaine, you're nodding. Anything to add?
Elaine: So most of the patients that are in our program are suffering from regular drug susceptible TB. But Nigeria does have a growing concern around multidrug resistant TB. I think that the data that we're collecting now, which tells us where we're finding TB cases, where the facilities are that are identifying these TB cases, where we've done outreach, social behavior, change interventions and what numbers we found as we did them all over Lagos, all over Kano. Where did we find the most cases? I think this is really important information for helping the Nigerian TB program to think through what they need to be doing proactively in the multi drug resistant TB disease prevention. Because where you get multidrug resistant TB is where you've had people who've had TB and it wasn't treated well and so it's come back and when it comes back, like all diseases, it comes back bigger and better.
Where do we need to be focusing our drug resistant TB efforts? Who are the providers most likely to see it? Because the truth is, we don't have the resources to train everybody on how to treat drug resistant TB. So particularly where you have limited resources, if you can use the data to help you target those resources better, so we train the right facilities and the right providers in the areas where we're likely to find drug resistant TB, that will go a long way in preventing a crisis that could still happen in urban cities in Nigeria.
Jason: Let me jump in on that, because I think that there's a great middle step in there, right? These programs and this infrastructure can collect a lot of information and you might say, okay, we have a lot of data coming in, and then you go to create a visualization or a map or a dashboard for it and you start to find that you have distinct gaps. That we have really good coverage in specific areas where we've gained public trust, where we've developed an easy-to-use app that providers are using and then you go to look at that larger scale and you think, oh hey, we have really good coverage right here, but we're not doing as well right here. From a public health perspective, that doesn't mean that that area may be at any less risk.
It could be that those areas are more at risk and that there's some of these other issues that have gotten in the way, that the providers haven't adopted them as readily ... That there's cultural sensitivities that have worked against us in trying to deploy different resources and opportunities there and I think that that's an important step that's often missed, using the dashboarding, using the custom data visualizations, using maps and those sort of analyses helps us sort of identify those gaps.
We can plot that out and kind of see where we know we don't have information versus if we have information and not knowing whether we have the right information, and it's definitely getting a necessary step in terms of being able to confidently hand something over to the government and say, "Here. This thing—stand up—it's ready for you." It’s being able to give them a fair assessment as to whether or not this is covering their population well.
Eric: Let's tie this up. We have a blank RFP Elaine. What does it say? How do we get all this under one roof?
Elaine: I wish I had a blank RFP. That would be great.
Eric: That's the beauty of the podcast.
Elaine: Yeah. There is actually going to be an RFP in Nigeria. It's going to be called the TB accelerator, it'll be funded by USAID. I think that what I'd love to propose is that for one, there are a lot of people now, there are several people, institutions now working on fixing this data collection issue, making it easier to collect data and easier to use data. There definitely needs to be some integration, streamlining, ensuring that all of these different systems speak to each other. That would be the first one. The second one is I think we have definitely not used enough data visualization. We do it a lot on our program and because we get to work with DSET.
Eric: For our listeners, DSET is? Or Jason, you want to describe DSET for everyone else?
Jason: Because I'm in DSET? Data science surveys and enabling technology division. It's the division that helps with data capture in all of its different forms and analysis and dashboarding and reporting and all things kind of data and technology. So we also do the website development and database creation on top of dashboarding and things of that sort.
Elaine: So we've been able to have this great partnership with DSET that allowed us to really maximize the use of data visualization on the program. It also allowed us to have our field staff become really competent at using things like Tableau and arcGIS so that they're doing it themselves in the field, not us doing it here and giving it to them.
And the data visualizations have been really powerful. They help us with our program management. They help us see where we've had impact and where we are missing information. There also are great advocacy tools.
And then the last thing is having processes to use these data on a regular basis. So I think having a requirement for very short cycle, short loop learning would be a great thing as well. And I think it should be a requirement in RFPs. And I think one of the great things about this program is it's taught us how to do that, which means we can take those processes and use them in other programs, whether it's environment or it's agriculture. So an RFP, I'd like to see it do more about having systems talking together, more about really using data visualization more, and then more of a requirement that we learn from our programs quickly and make changes quickly, where appropriate, so that we're not waiting months and months and quarters before we say, oh we have a problem. Let's go back and fix it.
Eric: And that speaks what Jason was saying about connecting those dots in the process. Would you agree Jason?
Jason: I would. I think all of those things are great to have in RFPs and I don't see them nearly enough. And also the other thing that I don't usually see enough in RFP's, I always sort of advocate at sort of the end of the program to get some individual assessments from people that have participated in the program, whether that's individuals that got screened for TB or were infected with TB and were going through that process, or providers surveys who are using these things before transitioning to the government or whomever's taking over this system at the end. I think setting that system up so that you can sort of maintain that feedback loop, because quite often what happens is we implement these things, they go out into the field and you don't have a mechanism to keep in touch with those same communities to make sure that it continues to work the way that it's intended, or that it's evolving or that it continues to be useful.
Eric: So I think you guys just covered the entire life cycle in perpetuity, which seems like a pretty good place to stop.
Elaine Baruwa: I agree.
Eric Tischler: And on that note, I want to thank you both for joining me.